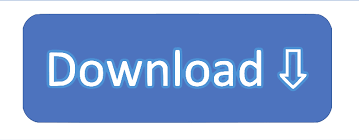
pedi - ‘diabetes pedigree function’ (a measurement I didn’t quite understand but it relates to the extent to which an individual has some kind of hereditary or genetic risk of diabetes higher than the norm). mass - body mass index ((weight/height)**2). insu - serum insulin (two hours after drinking glucose solution). #Crop devonthink pro skin
skin - triceps skin fold thickness in mm.pres - diastolic blood pressure in mmHg.
plan - the concentration of blood plasma glucose (two hours after drinking a glucose solution). preg - the number of times the subject had been pregnant. The attributes are as follows, and I list them here since they weren’t explicitly stated in the version of the data that came with Weka and I only found them after a bit of digging online: This dataset contains measurements for 768 female subjects, all aged 21 years and above. They have been heavily studied since 1965 on account of high rates of diabetes. The Pima Indian population are based near Phoenix, Arizona (USA). In what follows I’ll be mostly following a process outlined by Jason Brownlee on his blog. The simplicity made it an attractive option. The Pima Indians dataset is well-known among beginners to machine learning because it is a binary classification problem and has nice, clean data. In this (common) use case, it becomes a bit harder to say exactly why a certain prediction was made, though there are a lot of ways we can start to open up the black box. Some of this also has to do with the fact that when you train your model, you do so assuming that the model will be used on data that the model hasn't seen. In the above image, you can get a sense of how the earlier layers (on the left) are learning basic contour features and then these get abstracted together in more general face features and so on.
This has something to do with how neural networks work: you often have many layers that are busy with the 'learning', and each successive layer may be able to interpret or recognise more features or greater levels of abstraction. You put data in one end as your inputs, the argument goes, and you get some predictions or results out the other end, but you have no idea why the model gave your those predictions. A common criticism of deep learning models is that they are 'black boxes'.